5 Use Cases For Predictive Analytics in BI
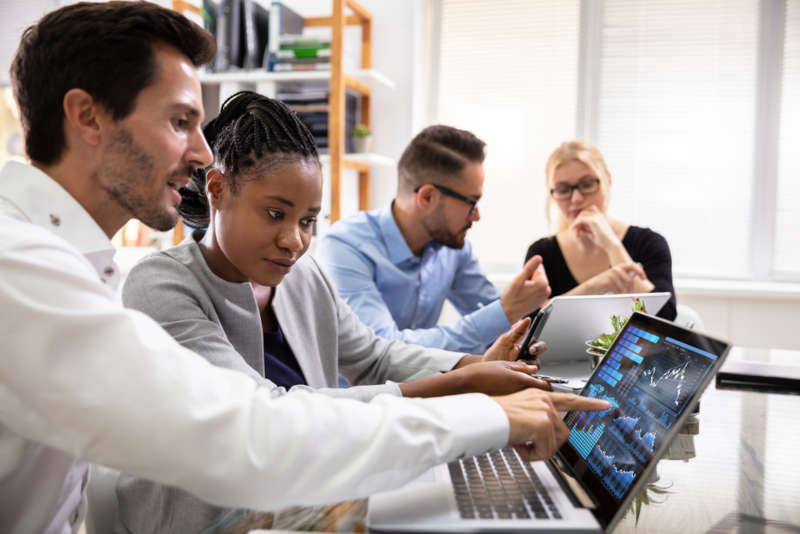
What do streaming service content recommendations, early illness detection in clinical settings, and sports predictions have in common? They all rely on predictive business analytics to identify future trends and patterns based on historical data. These examples only scratch the surface of predictive analytics use cases that can benefit various industries by generating actionable insights.
Predictive business analytics can enhance organizational success in several ways, from enhancing product development to improving customer retention.
What Is Predictive Analytics?
Predictive analytics is one of four types of analytics in the field of data analysis. Alongside descriptive, diagnostic, and prescriptive analytics, predictive analytics produce actionable insights for marketers and business leaders.
Specifically, predictive analytics assesses historical data, identifying trends and patterns from the past that may inform how a customer, industry, or market will act in the figure. Predictive analytics are also helpful beyond consumer spending, enhancing effectiveness in areas such as fraud detection, risk management, and predictive maintenance. Predictive analytics empower marketers and business leaders to make data-driven decisions based on forecasted trends by pointing out what may happen in the future.
An Overview of Predictive Analytics Models and Techniques
Predictive analytics use statistical techniques to analyze data to determine future outcomes. Artificial intelligence, data mining, machine learning, and modeling are all used in predictive analytics to detect patterns and use them to make predictions. While there are several predictive analytics models, the three most common are decision trees, neural networks, and regression.
Decision Trees
Considered the simplest of the predictive analytics models, decision trees organize data based on certain criteria. This organization style creates a data visualization reminiscent of a tree, with the branches representing possible outcomes and each leaf representing one specific choice.
Regression
The regression technique finds patterns in large datasets. Employed when data inputs have a linear relationship, regression determines a formula that best depicts the relationship between the data points.
Neural Networks
Designed to mimic the human cognitive process, the neural network model processes complex data relationships. As seen in uses such as artificial intelligence and pattern recognition, neural network modeling is useful when there is an overwhelming amount of data or a lack of useful formulas for a certain dataset.
Additional Predictive Analytics Models and Techniques
While decision trees, regression, and neural networks are the most commonly employed predictive analytics techniques, others also provide useful predictions. These additional techniques include cluster models, time series modeling, ensemble models, and classification models.
Request My Free DBA Program Guide
Predictive Analytics in the Marketplace
The best way to understand the uses and effectiveness of predictive business analytics is to consider various applications in the real world. Predictive analytics tools are enhancing the success of companies and organizations in industries such as healthcare, cybersecurity, retail, and supply chain manufacturing. Consider some powerful uses of predictive business analytics in the marketplace today.
Customer Lifetime Value
Spreadsheets and anecdotal data can only go so far in determining which customers are likely to spend money regularly. Predictive analytics tools remove the guesswork from the process, replacing it with clear insights that reveal which customers are most likely to return, which products or services they will most appreciate, and how to market to these customers specifically.
For example, the e-commerce logistics platform Parcel Perform saw a 40 percent increase in customer lifetime value thanks to Amazon Web Services analytics tools.
Healthcare Diagnosis
Predictive analytics models have become essential to the healthcare sector as the tools and techniques make positive use of the tremendous amounts of healthcare data generated each day. By analyzing patient histories and current illnesses, predictive analytics models help healthcare providers determine the best diagnoses and treatment plans for each patient. This advancement in technology has also empowered healthcare providers to treat patients earlier and providers have a better understanding of which conditions a patient may be at risk for acquiring later in life.
Home care platform AlayaCare employed predictive analytics to determine the likelihood of negative health events for senior citizens in their homes. The results were remarkable: AlayaCare reduced hospitalizations by 73 percent and emergency room visits by 64 percent among chronically ill patients.
Customer Segmentation
While companies have long marketed to various groups of people, their visibility into the data on those groups has been limited. With predictive business analytics tools, businesses can now understand their target markets based on data-driven organization. Predictive analytics models are useful for better understanding what motivates existing customer groups and learning about new potential markets.
PDI Technologies, a corporation that works with fuel and convenience businesses, witnessed the effectiveness of predictive analytics-powered customer segmentation when the company turned to the Oracle Cloud Infrastructure. Now, the company regularly rolls out data-driven loyalty programs, promotions, and partnerships based on customer insights—a venture so successful that PDI is currently the only gas station loyalty provider in the United States with two fuel brand customers.
Fraud Detection
As the uses of technology multiply daily, so do the security threats that companies face online. One of the most important cybersecurity tools available is fraud detection made possible by predictive analytics applications. By leveraging predictive artificial intelligence, businesses can reduce vulnerabilities in their systems. The predictive analytics applications rely on historical data regarding cyber-attacks and threats, notifying key individuals when similar behavior is identified.
In the case of a global electronics manufacturer, an analytics-based fraud detection and investigation tool led to immediate positive returns. A Kaizen anomaly detection solution with on-demand dashboards detected a fraud case in the first thirty days, achieving project payback in the first month of use.
Predicting Buying Behavior
“You don’t need to learn what customers say they want,” explains Cindy Alvarez, Principal Group Product Manager at Microsoft. “You need to learn how customers behave and what they need. In other words, focus on their problem, not their suggested solution.”
Predictive analytics tools applied to point-of-sale systems empower business leaders to do just that—understand how customers behave and determine what they need. Retailers can use data analytics to predict buying behavior by analyzing customer behaviors such as spending patterns, purchase history, and cart abandonment. Marketers can also use business intelligence tools to analyze if various customers are motivated to shop via social media platforms, emails, or text messages, which can help them predict where future sales will originate.
Tools that predict buying behavior can generate measurable positive outcomes for the businesses that use them. The History Channel, for example, leveraged predictive analytics technology to increase its customer acquisition by 500 percent.
Further Use Cases
There are many more examples of how predictive analytics are making a positive difference in the marketplace, such as:
- Churn prevention: assessing customer dissatisfaction to enable data-driven decisions that keep customers from leaving
- Next best action: analyzing buying patterns, customer behavior, and interactions to inform the best opportunities for reaching customers
- Volume prediction: determining patterns of increases and decreases in sales to facilitate streamlined workflows, processes, and staffing procedures
Additional use cases include content recommendations, risk modeling, quality assurance, and predictive maintenance.
Become a Business Intelligence Expert with a DBA in Business Intelligence from Marymount University Online
Corporations worldwide are looking for data-driven leaders equipped to apply predictive analytics tools to the challenges they face. The Online Doctorate of Business Administration in Business Intelligence at Marymount University will prepare you to leverage predictive analytics for positive outcomes in your company and career.
The fully online DBA in Business Intelligence features coursework in relevant technology. For example, Using Data for Business Intelligence includes coursework that covers:
- The technological and management aspects of using data to support advanced decision-making.
- How to use business intelligence to optimize business operations.
- Developing a data strategy for an organization of the student’s choice.
- Building a platform to create data-driven business intelligence.
- The phases of data exploitation including collection, storage, analysis, and visualization using state-of-the-art tools.
- Researching and applying artificial intelligence, robotic process automation, and other techniques to a business problem of the student’s choice.
In a course called Artificial Intelligence Applications, students:
- Study the application of artificial intelligence (AI) techniques in government and business transformation.
- Examines the role of various AI techniques in business intelligence.
- Learn about AI tools and techniques for implementation, including data science and machine learning technologies.
- Work with existing machine learning models and implement tools to support business intelligence using available data.
- Develop an AI strategy.
- Evaluate the competitive advantage and trustworthiness of AI.
The online DBA in Business Intelligence is a part-time, accelerated program that can be completed in as few as three years. A highly personalized interdisciplinary program, the online DBA empowers students to conduct original applied research directly related to their career goals. With no residency requirement and a cost-effective tuition investment, Marymount’s online DBA features supportive faculty experts and a concurrent dissertation model.
Become a BI leader with an online DBA in BI from Marymount University.