The Data Discovery Process Explained
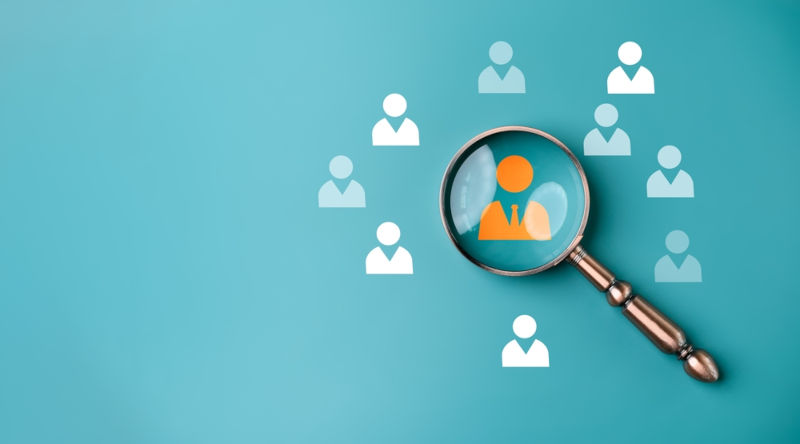
Multinational retail company Woolworths has over 200 stores, an online division, and vast customer and client data. But, like many companies, they needed more software to leverage that data meaningfully. Their system needed to be faster, easier to maneuver, and more accurate.
All of that changed when Woolworths implemented a new data discovery tool. The results were overwhelmingly positive: automated data scanning speed increased over 30 times. The company saved over a month’s working hours in a year. The software detects patterns and generates actionable insights through machine learning and artificial intelligence technology.
By leveraging all data streams available through data discovery, corporations like Woolworths achieve measurable positive outcomes for their customers, clients, employees, and the marketplace.
What Is Data Discovery?
The term “data discovery” refers to collecting and analyzing data from multiple sources to detect patterns, identify trends, and answer business questions. The data discovery process includes communicating insights drawn from data in visual ways that are accessible to non-technical users. With data discovery software, leaders and professionals in all types of roles can visualize, interact with, and understand relevant datasets.
Data Discovery Methods
There are several data discovery methods that illuminate where an organization’s data is stored and how it is processed. Traditionally, these methods have included:
- Data scanning: The process of examining digital files for data.
- Data mapping: The process of matching fields between two or more datasets.
- Data cataloging: The process of creating an organized data inventory.
With automated data discovery tools in place of these manual processes, organizations and professionals can access actionable insights through smart data discovery solutions.
Data Discovery Use Cases
Smart data discovery tools generate insights that empower leaders, managers, and all professionals to make informed decisions. There are dozens of use cases for data discovery tools in the real world, from demand forecasting to customer retention and churn prediction to personalized recommendations. Consider three such examples.
Improving Patient Outcomes
Leading pharmacy Walgreens generates tens of thousands of transactions every second. Each one represents hundreds of data points in essential categories like supply chain inventory and patient behaviors.
Their costly, slow data infrastructure made this data nearly impossible to understand and apply to decisions. So Walgreens turned to the Databricks Lakehouse solution. As a result, productivity has increased by 20 percent, which gives pharmacists more time to work with patients. Additionally, the tool has made innovative patient experiences possible, such as specialized services for terminal-stage breast cancer patients and convenience improvements that lower prescription wait time.
Identifying Opportunities for Cost Savings
South African aviation company Comair Limited operates up to 130 daily domestic and regional flights. Just a few years ago, the company relied on Excel spreadsheets to store and analyze data points ranging from pricing to flight punctuality. This system created data silos and an inability to understand how the data points related to one another. Meaningful insights were nowhere to be found.
Recognizing that a lack of infrastructure was the core of the issue, business intelligence leaders at Comair implemented Tableau to connect their data sources. The smart data discovery tool immediately pinpointed areas for significant cost savings. One insight shed light on an opportunity to save over $500,000 on fuel spending. Since fuel is Comair’s biggest expense, accounting for 23 percent of the company’s total costs, savings like these can significantly benefit the company.
Reducing Customer Churn
Vodafone Ukraine is the second-largest mobile phone in the country, which isn’t good enough for them. The company’s executives want to lead the corporation to the number one spot. Recognizing a gap in customer volume, the company turned to smart data discovery for analytics that could give them a competitive edge.
With SAS Customer Intelligence, Vodafone achieved a 30 percent reduction in customer churn. The company has also increased incremental revenue, reduced data processing times significantly, and increased the number of integrated communications from two to five.
Automated data discovery has enhanced Vodafone Ukraine’s opportunity to improve its market position in meaningful ways.
The Data Discovery Process
Data discovery is a repetitive process designed to help answer real business questions. This process can be broken down into five important steps:
- Goal setting
- Data preparation
- Data analysis
- Data visualization
- Iteration
Each of these steps is essential to the smart data discovery process.
1. Goal Setting
For data insights to be meaningful, they need to answer specific questions about an organization or its customers. That’s why the first step in the process is defining the goals or objectives for data discovery.
Consider the three case studies from earlier. Their respective goals may have sounded like this:
- We want to improve the patient experience at our pharmacies.
- We want to see where we are overspending.
- We want to leverage data to increase our customer base and gain a competitive edge.
A clear sense of problems that need to be solved and questions that need to be asked will help business leaders choose or participate in building the ideal smart data discovery tool. The right software solution will produce guided advanced analytics, which provides visibility into organization-specific data.
2. Data Preparation
The first component of data preparation is data ingestion: importing all the data an organization generates across various sources. This could include datasets compiled from transactions, customer interactions, or social media. Upon being brought to one place — ideally, a smart data discovery solution that can store, integrate, and analyze the data — the data is cleansed so that errors are eliminated and all data is formatted in an organized, uniform way.
3. Data Analysis
Once goals have been set and the data has been prepared, it’s time to detect trends, identify patterns, and produce insights. The data analysis phase seeks to answer the questions outlined in step one. Data discovery tools perform quick, comprehensive analyses by running specific queries that comb an organization’s datasets for relevant information.
4. Data Visualization
Rather than simply sharing data analysis insights in words or reports, the data discovery process includes presenting insights in accessible visual forms. Through dashboards, graphs, charts, and more, data visualization ensures that insights are easy to find, understand, and act upon to meet organizational goals.
5. Iteration
As organizations acquire more data minute-to-minute, repetition and up-to-date insights are imperative. Data discovery is not a one-time or static event. Instead, it’s a repeated process that leaders can adjust to answer arising questions, assess new datasets, and solve emerging problems.
Lead Data Discovery with an Online Doctorate of Business Administration from Marymount University
Organizations of all industries and sizes are leveraging the power of data discovery to meet their goals. The online Doctor of Business Administration (DBA) in Business Intelligence program at Marymount University equips professionals to lead in generating actionable insights for data-driven decision-making.
Courses such as Using Data for Business Intelligence, Maximizing Digital Transformation, and Artificial Intelligence Applications prepare data-savvy leaders for innovative careers.
Complete our 100 percent online, real-world-focused program in less than three years. Become a BI expert with a DBA in BI from Marymount University Online.